Impedance Mismatch | Data Science & Traditional IT Methodologies
Because this is a very broad topic, I’m writing this in the context of typical data driven businesses.
A paradigm shift occurs when people in a given field discard the ideas and rules that had been the basis for their entire way of thinking. Because of this aversion to change, paradigm shifts are generally difficult.
I think there is a general misconception of what a data scientist is. This causes confusion and counter-productive assumptions leading to bad decisions.
I recently read a post where a graduate engineer stated “isn’t all science data science?” WOW! Yes, all science is data science, it is, but, mainstream IT thinking or paradigms are generally not science based! This is an entirely separate topic unto itself.
It comes down to this, technology is starting to move towards science-based approaches when it comes to getting value from the data… Introducing the “Data Scientist”.
What is Data Science?
A Science based analytical approach being applied to computer data generated from various systems, databases and software applications.
Data Science is about, making observations, formulating hypothesis and then building experiments to prove or disprove that hypothesis (Null hypothesis).
Data Science is about applying strict scientific methods to discover unknown facts about data without introducing pre conceived notions into those experiments.
Data science differs completely from existing Decision support, BI and BA activities in that with data science, we don’t design into or bias the answers to questions as we do with traditional BI (this is not a negative, it just is). Data Science asks a lot of questions, formulates the hypothesis, creates experimental models and then proves or disproves those hypotheses by setting up various hypothesis testing scenarios. As a rule, no assumptions should ever be made during the process!
This approach bumps up against most traditional IT practices. Not hard to see why looking at this diagram.
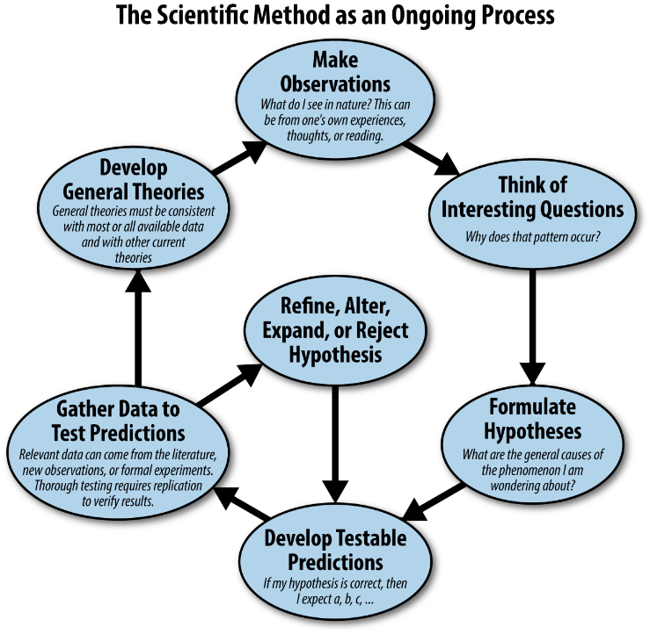
Figure: from Wikipedia
Where other approaches fail, Scientists use their skills and disciplined scientific approaches to discover otherwise unknown insights with these data. This is not a replacement for BI or traditional reporting it is a value-add proposition.
Data Mining
Some of these methodologies are also being used by data scientists today:
https://www.researchgate.net/publication/220969845_KDD_semma_and_CRISP-DM_A_parallel_overview
Helping your Data Scientist
It’s important to give your data scientists 3 key things:
- Lots of raw! data
- descriptions of your pain points
- Be prepared to answer a lot of their questions. It’s their job to ask a lot of questions about these data.
A day in the Life of a Data Scientist (labor intensive & no shortcuts)
Data Scientist will use tools such as Data Science Notebooks, Python and or R to start the exploratory data analysis activities to begin wrangling the data and documenting observations. Observations on the data is a highly detailed, challenging and time-consuming effort.
Typically, the many libraries used are numerous and involve wrangling various datasets and formats, de-duplication, de-nulling. Using libraries for Math, statistics, plots, documenting density, correlation observations, feature engineering, scaling features, data normalization etc. If there is no statistical significance or confidence indicator the data is sufficient to run an experiment the process starts over.
This all happens before building any prediction experiments, not to mention building production data science and model training/re-training pipelines.
The process involves many iterations of many iterations of many iterations.
Here is a little data science humor: A data scientist spends 90% of their time dealing with problems with the data and the other 10% complaining about the time they spend dealing with data problems.
But because of the science discipline, steps cannot be skipped, the exploratory data analysis EDA is critical step in the process.
Be nice to your Data Scientist
- Data scientists can be a tremendous value add that helps to find a wealth of information from data un-obtainable using other methods.
- Data scientists should not be constrained by traditional IT methodologies or approaches.
- Data scientists do not necessarily need previous “Business Domain knowledge” to do their job.
- Data Scientists are usually not a lazy lot (they work best with minimal supervision!)
- Data Scientists tend to be highly disciplined professionals.
What Skills Make up a Data Scientist?
Data Scientist are trained & educated in mathematics, science disciplines + strong programming skills.
The 8 Key IT Data Science Skills
- Scientific discipline (Relevant Education).
- Programming Skills.
- Statistics.
- Machine Learning.
- Mathematics, Multivariable Calculus & Linear Algebra.
- Data Wrangling.
- Data Visualization & Communication, plotting
- Software Engineering.
Conclusion
Data science is about bringing science to traditional IT data processing ala Advanced Analytics. As valuable addition to our data practices, Data Scientists, combined with BI and Data teams working together will deliver new opportunities and better insights into their data.
However, it is clear that data science is not a quick and dirty process that can be managed the way we traditionally approach development of software and BI Apps. Therefore, data science is not for everyone, businesses need to evaluate the true costs and benefits to investing in data science initiatives internally.